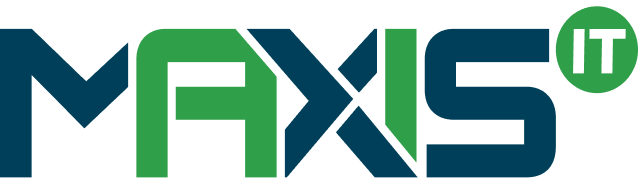
-
WHAT WE DO
- CLINICAL SOLUTIONS
- SERVICES
-
WHO WE ARE
-
RESOURCES
- CAREERS
- CONTACT US
What would you expect from a platform which offers to manage your clinical trials? Would you expect timely access to as authoritative, standardized and aggregated clinical trial operations data as well as patient data from site, study to portfolio level? Would you need efficient trial oversight via remote monitoring, statistically assessed controls, data quality management, clinical reviews, and statistical computing? Would you expect it to learn the patterns in the data and identify any discrepancies soon as they occur, in real-time?
If you don’t, it’s time you did. Yes, you heard that right. Demand more. Never settle.
Ensuring the Timely access to authoritative Data
After all, clinical trials are known to face issues with time and cost overruns. They could also fail to enroll on time, quality compliance, or low performance. Many even fail to clear the hurdles set by regulatory requirements. All this happens because the data was not accessible timely and required insight was not delivered to enable in-time decision making and issues were not identified or flagged in real-time and addressed appropriately.
Authoritative data is the sine-qua-non for any successful clinical trial. Only then would a trial stay within budget, meet its timelines and adhere to compliance requirements. There’s no disputing the fact that clinical data managers work very hard to ensure that their data is clean, but their task becomes superhuman as the data gets generated at tremendous rates and keeps on piling up. There’s always a risk of finding some gaps as some data usually goes missing. All these aspects make the task of a clinical data manager almost impossible to be done manually without the help of a tool.
How can technology help?
The market may be full of tools, but it’s important to ask if a tool has the required capabilities. It could help to improve the time taken to complete the task, beyond all recognition. It could aggregate all the clinical data in real time, across the data silos created by the disparate EDCs, CTMSs in use by the CROs and partners. It could offer a process powered by artificial intelligence and machine learning. It could go up the learning curve to quickly identify the trends in the data to flag any outliers and mitigate possible risks and manage the KPIs. It could offer actionable insights which support proactive action.
With the right technology, the data speaks to the mangers in various ways, like the following:
Who wouldn’t want to have a single tool which offers you all these capabilities, across your entire clinical trials portfolio?
Wake up call to Data Managers
Data managers need to refuse to be martyrs to their profession and be stuck with managing everything without the help of the right tool. Diversified host of eClinical systems only add to the issue, by creating siloed data and cannot offer an integrated view of all the trial data in real time. Technologically advanced tools like MaxisIT’s CTOS have been here for the last 17 years – with industry leading capabilities which make it possible for data managers to gain total oversight over their clinical trial portfolio data and enable them to take proactive action in real time. Data managers literally get into the driving seat when they adopt MaxisIT’s CTOS.
About MaxisIT
At MaxisIT, we clearly understand strategic priorities within clinical R&D, as they resonate well with our own experience of implementing solutions for improving Clinical Development Portfolio via an integrated platform. An ideal platform delivers timely access to study-specific as well as standardized and aggregated clinical trial operations as well as patient data, and allows efficient trial oversight via remote monitoring, statistically assessed controls, data quality management, clinical reviews, and statistical computing. Moreover, it provides capabilities for planned vs. actual trending, optimization, as well as for fraud detection and risk-based monitoring.
MaxisIT’s Clinical Trials Oversight System (CTOS) enables “data-driven digital transformation” by its complete AI enabled analytics platform. From data ingestion, processing, analysis to in-time clinical intelligence by establishing value of data. The CTOS empowers clinical stakeholders to mitigate risks and seize the opportunity in the most efficient manner at a reduced cost.
Clinical study designs are becoming increasingly complex. A growing number of studies are using adaptive designs and require decisions during the conduct of the study. At the same time there’s a growing demand for more amount of data, a larger variety of data types and time pressures on decision making. During clinical study conduct, scientists are under high time pressure as they need to manage a multitude of tasks, such as medical data review, signal detection on clinical study and project level, conducting preliminary analysis, preparing for database closure and working on publications and presentations.
Above all, clinical scientists are expected to drive innovation in pharmaceutical drug development with new clinical study designs, new assays and new ways to look at data. Innovative thinking requires time and a mind at ease, a contradicting requirement in the busy world of clinical studies. Since the evaluation of data is very often done with sub-optimal tools and processes requiring a lot of manual work, the clinical scientists have neither the time not space needed to turn creative and develop new ideas.
An effective and streamlined data flow from data capture to decision making can support the scientists in their intrinsic key responsibility to innovate drug development. The specific deliverables of an improved data flow must focus on two aspects:
Such improvements need to be achieved on the back of a high economic pressures for further improved operational efficiency and continuously high levels of data quality and regulatory compliance.
RETHINKING THE FLOW OF DATA
Addressing the scientists’ needs according to these requirements is a tall order. It requires a comprehensive approach looking at the systems, data standards and business processes in a combined fashion. Standardization is the underlying common characteristics to all of these because it offers re-usability and reduced time and effort.
Specifically, there are 3 topics that require consideration:
Finally, all functions involved need to be absolutely clear on their contribution and responsibilities across the entire data flow. In addition, there needs to be a clear distinction between mandatory process steps and deliverables versus areas where flexibility is possible and welcome.
SIMPLIFYING THE DATA FLOW AND TOOLS FOR CLINICAL STUDIES
The key design principles for the future system landscape was to minimize the number of tools and databases, eliminate redundant data storage where possible, and use the same tools or platforms across functions. The different options for the data flows needs to be reduced to one preferred way of working: on-line EDC data capture and access to clinical data via a graphical data review tool.
Tools and Platforms:
Data flow:
Data Standards
PROVIDING SPEEDY ACCESS TO STUDY DATA
A key requirement for clinical scientists is early and speedy access to study data. This can be greatly supported by the use of global data standards. A Gartner report showed that CDISC data standards can reduce the time for study setup by up to 80% and the time for data extraction into a usable format by up to 50%. Such time savings translate directly into the thinking time and space for scientists for decision making.
The redesigned data flow offers a variety of components for early and speedy access to study data.
STANDARDIZING DATA FORMATS AND DISPLAYS
Data standardization supports the fast database setup and enables a fast data flow during study conduct. Beyond that, standards are extremely valuable when it comes to integrated analysis reaching across studies. Finally, standards are a strong enabler for presenting data in an interpretable fashion. Downstream tools need to find variable names and types based on standardized names, and scientists are becoming used to this nomenclature.
For the re-designed data flow, CDISC data standards play a key role:
NEW RESPONSIBILITIES FOR CLINICAL SCIENCE
Early and speedy access to clinical data during study conduct is a privilege which comes with responsibilities.
In order to work with data, the clinical scientists need to acquaint themselves with the concept of data models. As a prerequisite to data exploration, the meaning and interpretation of the variables in data sets need to be understood.
When receiving data early during study conduct, it needs to be understood that the data are not clean. This should not cause friction in a team but should be understood by all parties involved.
Clinical scientists need to apply the concept of data exploration: first comes a question, then the data are explored using an adequate tool to get an answer to the question. Following the format of question and answer should help to look at data in a structured manner, without getting lost in a jungle of data.
CONCLUSION
The key elements to enable scientific innovation in drug development are
The daily transactions in drug development, however, frequently do not provide room for both, data availability and thinking time. Correspondingly, an improved data flow facilitated by an integrated data management platform coupled with data visualization tools can encourage innovation while maintaining overall efficiency and regulatory compliance.
About MaxisIT
At MaxisIT, we clearly understand strategic priorities within clinical R&D, and we can resonate that well with our similar experiences of implementing solutions for improving Clinical Development Portfolio via an integrated platform-based approach; which delivers timely access to study specific as well as standardized and aggregated clinical trial operations as well as patient data, allows efficient trial oversight via remote monitoring, statistically assessed controls, data quality management, clinical reviews, and statistical computing. Moreover, it provides capabilities for planned vs. actual trending, optimization, as well as for fraud detection and risk-based monitoring. MaxisIT’s Integrated Technology Platform is a purpose-built solution, which helps Pharmaceutical & Life sciences industry by “Empowering Business Stakeholders with Integrated Computing, and Self-service Dashboards in the strategically externalized enterprise environment with major focus on the core clinical operations data as well as clinical information assets; which allows improved control over externalized, CROs and partners driven, clinical ecosystem; and enable in-time decision support, continuous monitoring over regulatory compliance, and greater operational efficiency at a measurable rate”.
SDV is a very expensive process due to the time required to go through all the data at the various investigator sites. However, if we can target the patients and specify items the CRAs should look at when they visit a site, then the CRAs can spend more time looking at the important data but still spend less time overall. Although this may not be too useful for small studies, for large and mega-trials this can and has already saved millions. This approach is also being encouraged by the FDA and EMA as a possible method for improving the quality of trials and trial data. The key aspect here is defining the risk associated with a site, and how to change this over time.
CURRENT ISSUES WITH SDV
Quality risk management in clinical trials is often interpreted as risk elimination when it comes to SDV.
Pharmaceutical companies attempt to eliminate risk by performing 100% SDV. However, the cost of on-site monitoring is now around a third of the cost of a trial, so performing 100% SDV is a very expensive method of eliminating risk. Also, on-site monitoring involves many more tasks than just SDV. Tasks that are important for the overall quality and compliance, including GCP measures must also be performed.
The challenge for the monitor is then to perform 100% SDV and all the other tasks without spending a considerable number of hours at the investigator site. This leads to rushing the site visit, and thereby either missing issues, especially if they are related to cross checks between different questions on multiple pages, or not having enough time to clarify and explain how to avoid certain issues
RISK OF NOT PERFORMING 100% SDV
Studies have shown that only a very small percentage of data is changed due to 100% SDV, and the effect of this change on the primary analysis is negligible. Many of the data issues are identified and queried by Data
Management during screening rules checks and consistency checks. Therefore quality risk is not directly affected by 100% SDV. It was often thought that regulators preferred 100% SDV, and therefore not to do this may raise quality risk concerns.
However, papers from FDA (Guidance for Industry – Oversight of Clinical Investigations – A Risk-Based Approach to Monitoring, August 2011) and EMA (Reflection Paper on Risk-Based Quality Management in Clinical Trials, August 2011) have positively encouraged pharmaceutical companies to abandon the 100% SDV approach in preference to a more risk-based approach. So the risk of problems from regulators for following such an approach is now also no longer an issue.
WHAT IS A CENTRALIZED MONITORING RISK-BASED SDV APPROACH
Centralized monitoring can be thought of as moving a little bit away from the manual and subjective process to an automated and logical process. It is moving away from having humans look at and check the data to a process where all the data is checked by programs. As checks are then programmed centrally and run on all patient data, it then becomes possible to identify key data issues for the monitor to check, and therefore in effect ask the monitor to target their SDV and mostly check the data with issues.
This process has a risk associated with it, as the monitor is not performing all the checks manually, and they are not reviewing 100% of the data. However, the advantages of automating these processes far outweigh the benefits of the previous manual process. The process now becomes something like this:
1) Assign risk
Receive data from investigator sites
Receive feedback from CRAs.
Use programmed centralized checks to verify the data for consistency and accuracy as well as fraud.
Risk is assigned to each site based on the data checks, CRA feedback and knowledge from previous and ongoing collaboration.
What data of which patient the CRAs should check is identified based on the risk.
2) Data queries
Investigator sites receive queries raised by automated checks.
Submit centralized check programs regularly to monitor data quality of the latest data as an ongoing process.
3) Monitoring visits
If a site has some types of data issues coming up consistently, then inform the CRA to provide more training to reduce those issues
Inform CRAs about which patients to check and to which level for each site they are responsible for.
CRAs only check the data they are instructed to check.
CRAs then have more time to check:
ADVANTAGES OF A CENTRALISED RISK-BASED SDV APPROACH
The advantage of a centralized risk-based SDV approach is that systemic errors are easy to identify by looking at data trends and protocol violators. This means that if a site has misunderstood something this will become obvious.
It also means that all sites are being checked regularly by the automated checks on the latest data, and there is no need to wait until a monitoring visit is performed.
Automated programs also have the advantage that data errors, outliers, missing and inconsistent data are identified with logic rather than the luck of the eye, and more complex fraud checks and statistical analysis can be programmed very easily. Site characteristics and performance metrics can also be monitored over time by looking at high screening failure rates, eligibility violations and delays in reporting the data.
All this means that the CRAs have fewer data to review when they are at the site, which leaves them with time to both verify the source data and check the data to ensure it makes sense. They also have extra time to do more GCP and Process checks at the site, provide more training if required and so on. CRAs will then be able to visit sites with issues more often and spend a long time there and visits sites without issues less frequently. This all helps to improve the quality of the trial and the data.
This approach will not only increase the chances of identifying data issues, both random and systematic, it will also help to check for fraud and increase the quality of the trial. As more time will be spent on automatic checks, and less on on-site monitoring, the overall cost of on-site monitoring will be reduced. This saving will increase as the size of the trial increases from small to medium to mega-trials.
Today, cloud-based integrated platforms can assimilate source data and provide one source of truth for CRA’s to perform automated checks. With real-time visual analytics, these platforms make it easy to verify source data more effectively leading to improved site monitoring, better compliance and faster reporting of clinical data.
About MaxisIT
At MaxisIT, we clearly understand strategic priorities within clinical R&D, and we can resonate that well with our similar experiences of implementing solutions for improving Clinical Development Portfolio via an integrated platform-based approach; which delivers timely access to study specific as well as standardized and aggregated clinical trial operations as well as patient data, allows efficient trial oversight via remote monitoring, statistically assessed controls, data quality management, clinical reviews, and statistical computing. Moreover, it provides capabilities for planned vs. actual trending, optimization, as well as for fraud detection and risk-based monitoring. MaxisIT’s Integrated Technology Platform is a purpose-built solution, which helps Pharmaceutical & Life sciences industry by “Empowering Business Stakeholders with Integrated Computing, and Self-service Dashboards in the strategically externalized enterprise environment with major focus on the core clinical operations data as well as clinical information assets; which allows improved control over externalized, CROs and partners driven, clinical ecosystem; and enable in-time decision support, continuous monitoring over regulatory compliance, and greater operational efficiency at a measurable rate”.
CDISC standards have become an integral part of the life science industry; nevertheless, we will have to continue to deal with clinical data in different legacy formats for some time in the future. While the use of purely CDISC-formatted data from the very beginning of a submission project is unproblematic, combining data in legacy format with CDISC standardized data presents considerable challenges and therefore requires careful planning and special attention.
Scenario 1: “The files you sent are kaput“
XPT files cannot be opened in MS Word. This may seem funny but illustrates a challenge the industry is constantly facing.
Outside the clinical data science world, there is very little understanding of what needs to be done with clinical data for a regulatory submission to the FDA.
Regulatory Affairs departments are hesitant to approach the FDA outside the mandatory milestones. But with legacy data, it is important to contact the agencies with a sound data concept early to leave enough time for data preparation.
The pre-NDA / BLA meetings are usually too late for this discussion and should focus on important science aspects rather than data structures. Requests for the “full CDISC package” with a clean CDISC-Validator log often lead to some unnecessary effort.
Scenario 2: Analysis Datasets ≠ XPT Compliant
Starting Position – Data from multiple studies was analyzed using legacy formats. Dataset and variable names were too long for direct conversion to XPT format.
Possible Solution – Dataset and variable names need to be carefully renamed and standardized across all studies. Programs should be generated and submitted to map data back and forth between the data structures. Old and new names need to be documented in the DEFINE document.
Scenario 3: Comparing Original Results against Mapped Data Project Outline
For many projects only legacy raw data, legacy analysis data and original analysis results are available. Data preparation, analysis programs, and data definition documentation are missing. The customer needs a re-mapping of the legacy raw data to SDTM followed by the creation of CDISC compliant ADaM datasets. As a final QC step analysis results need to be recreated based on ADaM datasets and compared to original analysis results.
QC Result – We often see that discrepancies between the original and the re-programmed analysis emerge. Because of the lack of additional information on the original analysis, the resolution and documentation of findings is extremely time-consuming.
Potential Issues:
Scenario 4: Documentation
More often than not empty folders find their way into the folder tree. Sufficient documentation is key for reviewers to understand where the data came from and how it was processed.
Annotated CRFs and DEFINE documents are needed not only for SDTM data but also for legacy data.
Do not overload single documents. If more information is needed to understand certain aspects of the data, e.g. derivation of the key efficacy parameter, provide documents in addition to the reviewer’s guide and the define document, KISS — keep it short and simple and easy to understand
To Conclude
Every submission project is unique and needs careful planning to avoid costly delays
About MaxisIT
At MaxisIT, we clearly understand strategic priorities within clinical R&D, and we can resonate that well with our similar experiences of implementing solutions for improving Clinical Development Portfolio via an integrated platform-based approach; which delivers timely access to study specific as well as standardized and aggregated clinical trial operations as well as patient data, allows efficient trial oversight via remote monitoring, statistically assessed controls, data quality management, clinical reviews, and statistical computing. Moreover, it provides capabilities for planned vs. actual trending, optimization, as well as for fraud detection and risk-based monitoring. MaxisIT’s Integrated Technology Platform is a purpose-built solution, which helps Pharmaceutical & Life sciences industry by “Empowering Business Stakeholders with Integrated Computing, and Self-service Dashboards in the strategically externalized enterprise environment with major focus on the core clinical operations data as well as clinical information assets; which allows improved control over externalized, CROs and partners driven, clinical ecosystem; and enable in-time decision support, continuous monitoring over regulatory compliance, and greater operational efficiency at a measurable rate”.
Clinical organizations are under increasing pressure to execute clinical trials faster with higher quality. Subject data originates from multiple sources; CRFs collect data on patient visits, implantable devices deliver data via wireless technology. All this data needs to be integrated, cleaned and transformed from raw data to analysis datasets. This data management across multiple sources is on the critical path to successful trial execution, and submission.
DEALING WITH BIG DATA
SDTM data provides a powerful tool for cross study analysis, and can include various types of external data from labs, ECGs and medical devices. Wearable devices are becoming more popular, and can even be included in patient treatment regimens. Once confirmed, these data can provide fantastic insights into patient data and population health. This ‘big’ data can allow researchers to observe drug reactions in larger populations than those under study, and aligning with genetic data could even reduce wasted treatment cycles. In the digital age, our attitude to information is changing. The traditional model of data capture and supply, using an EDC system with multiple integrations has shifted downstream. Rather than being at the very center of this picture, EDC has shifted left slightly: companies now expect their clinical systems to act as a hub for all of the information relevant to their drug on trial, and are searching for a single source of the truth, whatever the data source.
DATA WAREHOUSING AND STANDARDIZED DATA
Growing volumes of data, global operations and increasing regulatory scrutiny are encouraging pharmaceutical companies and healthcare providers to develop Clinical Data Warehouses. Data warehouses can be a mine of information in a data-rich business environment, and can greatly enhance data transparency and visibility. The interoperability of systems is increasing along with interchange standards, and real world data is being collected more widely than ever before. Data warehouses are often used to aggregate data from multiple transactional systems. Such systems may have data structures designed for collection, and not be aligned with the reporting standard. Typically this data is transformed and then loaded into a central data model that has been optimized for analysis, for example, market research or data mining.
It is possible to design a Clinical Data Warehouse that follows the model of a traditional data warehouse with a single well-defined data model into which all clinical data are loaded. This can create a powerful tool allowing cross study analysis at many levels. Data is never deleted or removed from the warehouse, and all changes to data over time are recorded. The main features of a reporting standard must be ease of use and quick retrieval. SDTM is a mature, extensible and widely understood reporting standard with clearly specified table relationships and keys. The key relationships can be used to allow users to select clinical data from different reporting domains without an understanding of the relationships between domains. SDTM also allows users to create their own domains to house novel and as yet unpublished data types, so we can maintain the principles above for any data type, allowing powerful cross domain reports to be created interactively.
AUTOMATED DATA LOADING AND CONFORMANCE TO SDTM
Data may be loaded from the source transactional systems in a number of ways. With EDC, new studies are continually brought online, and may be uploaded repeatedly. Most warehouse systems include a number of interfaces to load data. Many also supply APIs to allow external programs to control the warehouse in the same way as an interactive user. A combination of robust metadata, consistent data standards and naming conventions can allow automated creation of template driven warehouse structures, and dedicated listener programs can automatically detect files, and automate data loading.
The SDTM table keys enable incremental loading, where only records changed in the source system are updated in the warehouse, saving disk space. We can also use the SDTM keys in our audit processing, and use them to identify deleted records in incrementally loaded data pools. SDTM conversion, data pooling at Therapeutic Area and Compound level, and Medical Dictionary re-coding can be handled automatically in the warehouse in the reporting standard. Use of SDTM facilitates the pooling of studies to the maximum version available, accommodating all of the studies in previous versions without destructive changes which would affect the warehouse audit trail.
Uses of a Clinical Data Warehouse include:
Each of these can deliver value to a customer, but each requires consistent data structures, in a format that can be easily understood by the warehouse consumers.
INTEGRATION AND RECONCILIATION OF SAFETY AND DEVICE DATA
A Clinical Data Warehouse may also be connected to a transactional Safety system. This, coupled with the SDTM data warehouse can allow reconciliation of the two data sources, a crucial task as the Clinical studies are locked and reported. Automated transformations can account for the different vocabularies in the two systems, and the records can be paired together in a dashboard. The dashboards themselves can be configured to highlight non-matching records, and also to allow data entry to track comments, and acceptance of insignificant differences. Reconciliation involves both the Clinical and Safety groups, but could also be carried out by CRO users responsible for the studies. This enhances collaboration between the sponsor and CRO, and provides an audited central secure location to capture comments. Security is paramount in an open system, and the warehouse’s security model is designed to allow CRO users to only see the studies they have been assigned to, hiding other clinical studies from the dashboards and selection prompts. As a serious adverse event must be reported within 24 hours, it is possible that that event could be reconciled against the clinical data the following day. MHealth data can be integrated automatically using the IoT Cloud service, with patients automatically enrolled into an EDC study. This can be reconciled with CRF data and automatically loaded to the Business Intelligence layer.
TO CONCLUDE
SDTM can be of huge benefit to the users of a Clinical Data Warehouse system, allowing data pooling for storage, audit and reporting. Use of data standards has already transformed Clinical research. The next generation of eClinical Software should place those standards in front of programmers, inside the tools they use every day, and allow them to automate transformations to and from review and submission models, respond quickly to regulatory inquiries on current and historical data, generate automated definition documents and support a wide range of data visualization tools. Study component reusability and automatic documentation together enable clinical organizations to have greater clarity on what has been done to get from source (e.g. EDC, labs data) to target (e.g. SDTM) – to turn on the light in the black box.
Ultimately, leveraging standard, re-usable objects accelerates study setup, and combined with automation reduces manual processes, and increases traceability.
MaxisIT’s Clinical Development platform integrates a best in class data management platform, allowing clinical trial sponsors to automatically load and control data from EDC and various external sources, transform this from the collection standards into SDTM without user input, and provide the SDTM data to dynamic, near real-time analyses which can be compiled into internet-facing dashboards.
About MaxisIT
At MaxisIT, we clearly understand strategic priorities within clinical R&D, and we can resonate that well with our similar experiences of delivering Patient Data Repository, Clinical Operations Data Repository, Metadata Repository, Statistical Computing Environment, and Clinical Development Analytics via our integrated clinical development platform-; which delivers timely access to study specific as well as standardized and aggregated clinical trial operations as well as patient data, allows efficient trial oversight via remote monitoring, statistically assessed controls, data quality management, clinical reviews, and statistical computing.
Moreover, it provides capabilities for planned vs. actual trending, optimization, as well as for fraud detection and risk-based monitoring. MaxisIT’s Integrated Technology Platform is a purpose-built solution, which helps Pharmaceutical & Life sciences industry by “Empowering Business Stakeholders with Integrated Computing, and Self-service Dashboards in the strategically externalized enterprise environment with major focus on the core clinical operations data as well as clinical information assets; which allows improved control over externalized, CROs and partners driven, clinical ecosystem; and enable in-time decision support, continuous monitoring over regulatory compliance, and greater operational efficiency at a measurable rate”.
Metadata enables exchange, review, analysis, automation and reporting of clinical data. Metadata is crucial for clinical research and standardization makes it powerful. Adherence of metadata to CDISC SDTM has become the norm, since the FDA has chosen SDTM as the standard specification for submitting tabulation data for clinical trials. Today, many sponsors expect metadata to be not just compliant to CDISC but also to their own standards. Creating metadata that is consistent and accurate at every point of time from setup until and after the database lock remains a challenge for operational clinical data management. Metadata repositories help in creating standardized metadata but it is just the beginning and there is a need for more.
THE NEED FOR QUALITY METADATA
Metadata is defined to be data about data, but is it that simple? No, there is much more to it and more so in the clinical world. Clinical metadata provides conceptual, contextual and process information which not only defines data but also gives insight into the relationship between data. Metadata enables exchange, review, analysis, automation and reporting of clinical data. Standardization helps exchange and use of metadata across different processes during the life cycle of a clinical trial at the conceptual level but there is a need for flexibility at the contextual level. The context is dynamic. Metadata Repositories (MDRs) address standardization at the conceptual level. Leveraging flexibility at the contextual level is what makes metadata more meaningful and usable. While it is clear that metadata is crucial to create high quality clinical databases, achieving high quality metadata continually remains a challenge for clinical data management.
CHALLENGES ON THE ROAD TO QUALITY METADATA
How do we make sure trial metadata is consistent with CDISC SDTM standards? What if the sponsors have their own standards and are actively involved in the review? How do we balance the diverse sponsor needs? How do we keep up with standards that are changing constantly? How do we make sure that the trial metadata is both accurate and consistent? And how do we do it efficiently and effectively, saving both time and costs? While all of these continue to be the major questions that need to be addressed at the conceptual level, they give rise to many more questions that need to be addressed at the contextual level. These questions trickle down to the role of a programmer who has to find answers and make day to day decisions to provide quality metadata. Most of the questions have been discussed and addressed quite often at the conceptual level suggesting the metadata driven approach and need for seamless integration of processes and people. But what do they mean for a programmer and how do they translate into day to day tasks for a programmer who actually creates the metadata? I would like to focus on and draw attention to the questions that arise at the contextual level and discuss a few scenarios a programmer is confronted with on a day-to-day basis while creating the clinical metadata.
Access to Metadata in Real-time
Today, sponsors are actively involved in the review of the clinical databases and expect high quality databases and metadata. Sponsors have their own checks for validating compliance which are run on every snapshot and expect no output. Since databases are set up in a test environment and with test data, quality metadata would translate to metadata that is consistent with the current data, which is test data. And the moment we go live, we are expected to provide metadata that is consistent with the live data. Most of the time, a snapshot of the database with accurate metadata is expected to be available on the day we go live. How do we make this possible given the time constraints? And it doesn’t stop there. Live data changes every day, and the snapshots sent to sponsors should always be consistent and compliant, which requires them to be accessible in real-time.
Standards that change
New versions of standards contribute to overall improvement of quality and broaden the scope of domains. New versions are ‘nice to haves’ and sponsors will always want them implemented. Upgrading to the latest standards while the clinical trial is ongoing and the database is already set up brings in challenges. Upgrading to the latest standard doesn’t just mean copying the latest version of the metadata standard from the MDR. Since all of the contextual metadata for the trial is set up, a programmer would aim to retain it where applicable and make the upgrades only where needed. How do we do this given the time and cost constraints? How do we achieve compliance both with the standards and the trial in such cases and also be efficient?
Conflict of Standards
Standards are changing and just when we think we have figured out mechanisms to cope with changes, we are confronted with the discrepancies between standards, discrepancies between sponsor and CDISC standards, and discrepancies between ‘the’ standards. One such example would be: Dataset Column length requirement by the FDA. We have all seen the ‘Variable length is too long for actual data’ error on Pinnacle21. Compliance is always questioned when there are discrepancies. Discrepancies as such need to be reported and addressed within very short frames of time and with a rationale. It is not easy to convince sponsors to ignore a Pinnacle21 error.
Non-DM datasets
Datasets that are not generated by data management but are part of the submission package are Non-DM datasets. Datasets that are not part of the database when it is set up but are part of the submission package are to be dealt with for most clinical trials. Examples of such datasets are PC, PD, PP and so on. It is the responsibility of the programmer to make sure the metadata for all these datasets is complete and consistent. In case of blinded trials, these datasets are only delivered on the day of lock. Having such Non-DM datasets added to the rest of the datasets and delivering accurate metadata for these datasets on the day of lock is quite a task. What makes it difficult is the fact that you get to see the datasets for the first time on the day of lock when we are always running short of time and finding issues that need to be fixed right away. These datasets only add to the pressure. How can the consistency be checked for in such cases when the datasets are not part of the database and you cannot run all those checks which you would otherwise run on your database against the standard repositories? Will validating the datasets and running Define.xml on Pinnacle21 suffice? These are some of the few scenarios, every programmer encounters while a clinical trial runs its course. These happen to be more critical for early phase trials where trials last for very short periods of time and need to go through all of the workflows any other trial would but at a pace that is 10 times faster. Everything here needs to happen ‘on the go’ without compromising on quality.
IS QUALITY METADATA CONTINUALLY ACHIEVABLE?
To achieve quality metadata continually, MDRs or Metadata repositories should be generic, integrated, current, and historical. In order to accommodate the variety of sponsor needs, hierarchical MDRs need to be implemented with focus on standardization and reuse. The hierarchical nesting should be in the order of CDISC SDTM, SPONSOR/SGS STANDARD, THERAPEUTIC AREA, and CLINICAL TRIAL METADATA. This would be the first step to creating and providing sponsors with quality metadata that is consistent with both standards and clinical data right from the setup until and after the database lock and thus accurate at every point of time.
About MaxisIT
At MaxisIT, we clearly understand strategic priorities within clinical R&D, and we can resonate that well with our similar experiences of delivering Patient Data Repository, Clinical Operations Data Repository, Metadata Repository, Statistical Computing Environment, and Clinical Development Analytics via our integrated clinical development platform-; which delivers timely access to study specific as well as standardized and aggregated clinical trial operations as well as patient data, allows efficient trial oversight via remote monitoring, statistically assessed controls, data quality management, clinical reviews, and statistical computing.
Moreover, it provides capabilities for planned vs. actual trending, optimization, as well as for fraud detection and risk-based monitoring. MaxisIT’s Integrated Technology Platform is a purpose-built solution, which helps Pharmaceutical & Life sciences industry by “Empowering Business Stakeholders with Integrated Computing, and Self-service Dashboards in the strategically externalized enterprise environment with major focus on the core clinical operations data as well as clinical information assets; which allows improved control over externalized, CROs and partners driven, clinical ecosystem; and enable in-time decision support, continuous monitoring over regulatory compliance, and greater operational efficiency at a measurable rate”.
CDISC defines SEND as an implementation of the SDTM standard for nonclinical studies. SEND specifies a way to collect and present nonclinical data in a consistent format. SEND is one of the required standards for data submission to FDA. SEND = Standard for the Exchange of Nonclinical Data.
Sponsors are currently focused on processes and tools to receive, transform, store and create submission-ready SEND datasets. Their decisions and implementations are presently driven by the nature and variability of their data sources, understanding of SEND, determining how best to prepare and generate SEND datasets that will successfully load into NIMS, and putting in place quality assurance and data governance controls. These are key foundation steps in any implementation. Let us understand SEND compliance better through SEND implementation objectives, stakeholders, requirements, challenges, and opportunities.
SEND objectives
Sponsors need a shared vision for how SEND implementation will improve their R&D operations. More than implementing a new data format, it should:
SEND stakeholders
Here is a list of all clinical study stakeholders involved in SEND dataset creation and compliance.
Sponsor requirements
CROs can’t implement all SEND compliance requirements, even for sponsors outsourcing 100% of their nonclinical studies. Here are the requirements.
New elements of the sponsor’s study workflow include mapping internal LIMS data extracts to SEND data model, integrating external CRO datasets, dataset versioning and error handling, validation and submission of integrated datasets to FDA, creating Define Files, Validation Reports, and Study Data Review Guides and coordinated response to FDA queries across Study Reports and SEND datasets
Implementation challenges
SEND implementation is more complex and time-consuming than most sponsors expect! Here are a few of the challenges.
Implementation opportunities
The Potential efficiencies arising from SEND compliance include higher initial data quality and fewer protocol amendments with early access to interim datasets. Other benefits include automated dataset integration and versioning, automated study table generation, expedited FDA review and faster response to review questions
As the industry’s experience evolves, it is clear that sponsor implementations will extend into study planning and preparation stages, and expand the needs of their processes and tools to support submission requirements. It is only then that they will likely step into the realm of how they themselves can routinely consume and use standardized data in research and development activities.
MaxisIT
At MaxisIT, we clearly understand strategic priorities within clinical R&D, and we can resonate that well with our similar experiences of implementing solutions for improving Clinical Development Portfolio via an integrated platform-based approach; which delivers timely access to study specific as well as standardized and aggregated clinical trial operations as well as patient data, allows efficient trial oversight via remote monitoring, statistically assessed controls, data quality management, clinical reviews, and statistical computing. Moreover, it provides capabilities for planned vs. actual trending, optimization, as well as for fraud detection and risk-based monitoring.
Moreover, it provides capabilities for planned vs. actual trending, optimization, as well as for fraud detection and risk-based monitoring. MaxisIT’s Integrated Technology Platform is purpose-built solution, which helps Pharmaceutical & Life sciences industry by “Empowering Business Stakeholders with Integrated Computing, and Self-service Analytics in the strategically externalized enterprise environment with major focus on the core clinical operations data as well as clinical information assets; which allows improved control over externalized, CROs and partners driven, clinical ecosystem; and enable in-time decision support, continuous monitoring over regulatory compliance, and greater operational efficiency at a measurable rate”.
The drug development industry and regulatory agencies continue to struggle with implementing CDISC for both the study workflow and the submission review process. Several factors contribute to these ongoing challenges including limitations within the CDISC standards themselves and the inability to represent complex relationships across clinical information in limited tools such as Excel.
In our personal lives we live in a connected world where all our information is linked together (e.g. Facebook, LinkedIn). We take the availability of information for granted and don’t realize what’s under the covers which links that information together. If you search for information about any disease a family member has, e.g. Alzheimer’s, you receive a LOT of interconnected information which helps you understand more about the disease and make better decisions about your family member.
Information within our clinical trials has the same dynamic relationships but unfortunately, we store our standards and data in just 2 dimensions with no robust way of linking that information.
THE PROBLEM – Separate data sets with variables and values.
The real problem lies in trying to pull this information together in a meaningful and clinically relevant way for a clinician who is trying to reach a conclusion. The problem arises when these connected and interrelated data points become disconnected and unrelated when we try to represent them.
Whereas technologies such as Google and Facebook integrate these relationships inside their data, in our world the institutional knowledge in our heads is what connects the data points. There are no electronic links between the data and nothing that really provides traceability likes everyone claims.
Our industry provides ‘specifications’ or ‘metadata’ that supposedly describe what our data will look like. But neither of them actually interacts with each other! This gives us this false sense of traceability or compliance that because we are checking the box saying we have specifications, we have better quality data. In reality, we are fooled into believing that our ‘specs’ give us quality data.
CONNECTING THE DOTS
The first step in connecting the dots is for the industry to stop using the word ‘metadata’. Most industries out there are not even sure what the word metadata means or use it in a very different context. The reality is that all the information we collect whether it is the value of a blood pressure reading, the name of a variable, or the length of numeric value is all data. Data that must be linked together in intelligent ways to really allow us to use our data.
We can connect this information in the form of a graph. The graphs I am talking about are the graph structures used by databases for semantic queries with nodes, edges and properties to represent and store data. A key concept of the system is the graph (or edge or relationship) directly relates data items in the store and the relationships allow data in the store to be linked together directly, and in many cases retrieved with one operation. This contracts a relational database. By baking the relationships into the database, institutional knowledge is not needed to connect the dots.
Our industry continues to struggle due to the limitations of the underlying models and the current technology used by industry to describe the multi-dimensional nature of clinical information. We will continue to struggle if we don’t look to embrace new ways of modeling our clinical information and really answering the questions we have in the clinical development process.
In our personal lives, we live in a connected world where all our information is linked together (e.g. Facebook, LinkedIn), yet we don’t take that simple step of realizing how we could represent the information in our clinical research world in the same way. In conclusion, we should stop trying to build ‘traceability’, ‘governance’, or ‘linkages’ in a world where the underlying models and existing technology can’t support it.
Patient Journey
It is important for pharmaceutical companies to understand the journey of the patient through the care pathway. They should understand the relevant patient population, the most relevant comparators and the potential drivers of effectiveness, which may create a gap between efficacy and effectiveness.
The key to mapping a patient’s journey in clinical trials is through documentation. Although various data capture systems support the clinical development, these systems are not typically connected to each other, thus making manual processes and individual data entry necessary. Ultimately, all information for the approval of a new pharmaceutical product is submitted electronically in one document. This creates an unusual situation, in which data are still recorded on paper documents but are also available online. For e.g., take the background of a patient which can often be found in the CTMS, the carrier, and IRT system. All of these systems receive the address via data upload – either from a list or by manual entry, both of which create the possibility of error. The job of a patient data repository is to connect such patient data stored in different systems like the CTMS, IRT, etc. and to enable optimal utilization for further analysis or documentation. Here are the advantages of using a patient data repository:
The adoption of a patient data repository has the potential to offer researchers a complete picture of the drug development journey – from manufacturing to the patient – with appropriate oversight and support.
About MaxisIT
At MaxisIT, we clearly understand strategic priorities within clinical R&D, and we can resonate that well with our similar experiences of delivering Patient Data Repository, Clinical Operations Data Repository, Metadata Repository, Statistical Computing Environment, and Clinical Development Analytics via our integrated clinical development platform-; which delivers timely access to study specific as well as standardized and aggregated clinical trial operations as well as patient data, allows efficient trial oversight via remote monitoring, statistically assessed controls, data quality management, clinical reviews, and statistical computing.
Moreover, it provides capabilities for planned vs. actual trending, optimization, as well as for fraud detection and risk-based monitoring. MaxisIT’s Integrated Technology Platform is a purpose-built solution, which helps Pharmaceutical & Life sciences industry by “Empowering Business Stakeholders with Integrated Computing, and Self-service Dashboards in the strategically externalized enterprise environment with major focus on the core clinical operations data as well as clinical information assets; which allows improved control over externalized, CROs and partners driven, clinical ecosystem; and enable in-time decision support, continuous monitoring over regulatory compliance, and greater operational efficiency at a measurable rate”.
As the coordinator or manager of a clinical trial, you will have one simple goal: You would want your clinical trial to be successful. After all, it is your responsibility.
However, to guarantee success, you need to be able to manage the project efficiently.
It is of prime importance that, at any time during the project, you have the ability to get a good overview of the progress of the study; that you can plan tasks, activities, and responsibilities; that you can track and monitor any action; and that you can easily organize all clinical study relevant documents and information.
But what system can make all these possible?
Are simple lists generated in Excel sufficient for recording all relevant information and documents? Are the lists clear and well-structured to control the development of my clinical study? Should I implement a Clinical Trial Management System (CTMS)?
If considerations like leave you with a sense of déjà vu, then you are in the right place to get some answers.
After all, the devil is in the detail!
It is important to know which information we need to record for reliable planning and monitoring of tasks and activities in managing clinical trials. We can only make the right decision if we are aware of the requirements.
Unfortunately, this decision is often made in favor of an MS Office package (or comparable programs) without any serious consideration. I can understand that people are used to working with these applications on a day-to-day basis and with the help of these programs it is easy to come up with an overview list in a short time.
The real problem, however, lies in listings that can get out of hand quickly. As a result, there would be a plethora of documents and their different versions. Such a decentralized organization of information in multiple listings frequently leads to great complexity and confusion.
“Which version of which document shows me the truth?”
To make things worse, lists of different versions or revision status could be in circulation. You would soon be wondering which version of which document shows you what you need to know. In the worst case, members of the study team waste precious time working on the basis of divergent and/or not up-to-date information.
“Why does it look completely different on my screen?”
The completely different look syndrome stems from simple technical issues like incompatible program versions being installed by different users. If you are running a complex trial with an international study team, simple lists probably will result in disappointment and confusion in the long run.
So what is the alternative solution? Can a Clinical Trial Management Software (CTMS) help to improve the working routine for you and your study team? The answer is, a qualified yes.
I qualify my statement because the implementation of such a system calls for some additional effort. Also, in the beginning, not everyone will be thrilled to leave their “comfort zone MS-Office”. However, in my experience, it is worth the investment as the CTMS comes with the following strengths and benefits:
If a CTMS is so capable, then why is a Clinical Trial Oversight Software (CTOS) necessary?
Well, to tell you the truth, the ‘M’ for Management in CTMS is no longer applicable to large scale clinical trials. Clinical trials spanning geographies often work with several CROs to conduct proceedings. These CROs have a management system of their own where they hold and manage the clinical trial data. With several CROs comes the problem of more than one CTMS. These disparate systems do not talk to each other. As summarized in this article, the important thing for the sponsor to do is oversee and not manage. That brings us to the CTOS.
Why Clinical Oversight?
Starting from the protocol development stage to IND/NDA submission to Regulatory authorities, the basic idea of clinical trial oversight is the identification of the risks on a continuous basis for all risk‐bearing activities throughout the clinical trials. This risk identification is done on the basis of existing and ongoing or emerging information about the investigational product(s).
By applying risk-based quality management approaches to clinical trials one can facilitate better and more informed decision making while making the most of the available resources. It is a prerogative of all involved parties to contribute to the delivery of an effective risk‐based quality management system.
How different is a CTOS?
A significantly large percent of sponsors and CROs still rely on manual processes and spreadsheets even though they probably have some sort of a CTMS in place. They are forced to extract data from different systems, so ultimately, they’re pulling everything together in spreadsheets and other manual tools in order to get a full-picture view.
What sets advanced trial oversight solutions apart is their ability to bring together all of the trial data (from all spreadsheets, data contained in different CTMS of CROs) all in one place without a big investment. Be it risk-based monitoring, data-driven enrollment decisions, or other data-driven mechanisms, the individuals involved in the study have a single place to go for that information. It’s a more efficient way to manage things.
Implementing a CTOS will offer you a number of significant advantages, listed below.
Faster trials: CTOS will equip study teams with easy to use role-based dashboards and streamlined navigation that will improve productivity and speedy trial execution.
Better decision making: It will also enable proactive closed-loop issue management and improve strategic trial planning with a complete real-time view of trial status.
Streamlined clinical operations: Finally, a CTOS will provide one seamless system of record for shared CTMS, TMF, and study start-up content, improving efficiency and streamlining operations.
What does the future hold?
Speaking from a process and a technology standpoint, the industry is going to see sponsors and CROs start to work more collaboratively together because both parties will need access to integrated data in at least near real-time. Emerging technologies hold out a promise to be key enablers for both the formulation and execution of strategy. To be prepared for what’s coming, organizations need to look at their entire process first and find the hidden manual processes – and eliminate them. They can overcome their inefficiencies by implementing technology solutions that enable transparency, agility and anticipatory oversight with the able support of technology like MaxisIt’s Clinical Trial Oversight Software (CTOS).
MaxisIT
At MaxisIT, we clearly understand strategic priorities within clinical R&D, and we can resonate that well with our similar experiences of implementing solutions for improving Clinical Development Portfolio via an integrated platform-based approach; which delivers timely access to study specific as well as standardized and aggregated clinical data, allows efficient data quality management, clinical reviews, and statistical computing.
Moreover, it provides capabilities for planned vs. actual trending, optimization, as well as for fraud detection and risk-based monitoring. MaxisIT’s Integrated Technology Platform is a purpose-built solution, which helps Pharmaceutical & Life sciences industry by “Empowering Business Stakeholders with Integrated Computing, and Self-service Analytics in the strategically externalized enterprise environment with major focus on the core clinical operations data as well as clinical information assets; which allows improved control over externalized, CROs and partners driven, clinical ecosystem; and enable in-time decision support, continuous monitoring over regulatory compliance, and greater operational efficiency at a measurable rate”.
Things seem to be set in motion in clinical trial management when programmers ready to perform the analysis get their code-happy hands on the clinical study data – however, once the CROs drop the data off, how does it actually make the journey to the statistical programmer’s desktop? This is a critical process, but too often compressed deadlines result in fragmented systems built with a collection of one-off programs that tend to become complicated over time and the data workflow lifecycle becomes fragmented. This article discusses the challenges of supporting data from multiple vendors including the issues of not using a consistent framework. It goes on to discuss the benefits of a common platform, breaking clinical data management activities down into “actions” – and driving those actions with object-oriented metadata and rapidly building capability through automated processes.
The problem with outsourced data collection
Most clinical organizations receive data from outside vendors and prepare this data for statistical processing. Whether as a consequence of dealing with multiple outside vendors or due to the specialized needs that differentiate the underlying science within one study from another, the format of the data delivered by the CROs will vary (one vendor delivering SAS datasets, another delivering Excel spreadsheets) as will the preparatory activities that ready the data for analysis (like using passwords to extract a zipped archive, or applying coding and edit checks).
Another element complicating this ecosystem is the organizational structure of business. Inevitably, the strategic responsibility for defining the systems within the company will be jointly shared by functional groups, such as between informatics and IT.
To receive data from CROs, a system exposed to the outside world through the corporate firewall will exist (likely managed by IT) and data consumers (like informatics) will have to use these shared systems rather than build their own.
Many organizations lack a standard operating system and operate in a mixed environment using UNIX, Linux, Windows, and various file servers. A formidable enterprise consideration is scalability, manifested as the ability of the system to accommodate changes for future business without disrupting ongoing or legacy activities.
Speaking in the language of an applications architect, the system must:
Chaotic Approaches to Defining Workflows
Many organizations function on a day-to-day basis within the chaotic environment of getting data from multiple vendors into the analysis pipeline. Perhaps when the group had to manage data for only a study or two it was possible to write unique programs for each workflow. As the organization begins to support more studies and concurrently more data, the one-off program approach becomes less ideal.
As people leave the company or move on to other roles, the knowledge about how to maintain the systems is lost. Fewer and fewer people are capable of fixing problems within each data flow, meaning there are numerous single points of failure within the clinical data management lifecycle where communicating program failures and quickly implementing solutions becomes quite taxing.
Over time, the tidy collection of custom programs dealing with one or two studies evolves into an unmanageable assortment of code and scripts. Challenged to support multiple legacy workflows and new business, the staff finds itself running around in circles trying to overcome breakdowns in the system just to keep the business in motion. Some organizations feel this pain profusely having long outgrown the ad-hoc approach to clinical data management; other organizations are at the early stages of growth and are realizing the need for something more robust while the pain is still manageable.
Automation through Metadata
Metadata is a nebulous term, one overused in the industry. In the context of this article, metadata is taken to mean the elements of data management that differentiate processes, and therefore enable automating workflows described as a sequence of reusable actions driven by externally captured metadata. The important thing to focus on is the flexibility of the technology to allow you to define and group metadata in a valuable way. A metadata ontology or syntax needn’t be too verbose, it simply needs to provide a vehicle to define data elements and their values. The system can interpret these values in a way that is helpful.
The art lies in determining the proper tradeoff between automation and flexibility. Certain elements of the system are inevitably not going to be standard – for example, some vendors may provide data that requires transposition. The system can support custom tasks while still preserving consistency by capturing the metadata about the locations and names of custom programs being run. This provides insight into the “unique” pieces of the system over time and potentially offers an opportunity to gain additional efficiencies by integrating those features as they stabilize. At a minimum, at least the framework maintains a “single source of truth” regarding the unique components of each flow and the standard components of each flow.
Metadata is one of those buzz-words that gets thrown around in a number of contexts and takes vastly different meanings. If the business had needs that could benefit from a metadata-driven solution, it is highly possible to build these needs into any re-design without forcing a technology or product choice. As an example, the normal ranges check and unit conversions are metadata driven processes. By associating a specific type of data transformation with a column of a table or row of data, the system could easily capture the calculation lifecycle for given data points.
Likewise, study level metadata such as the name of the trial could easily be associated with studies. Processing level metadata (such as the location of directories) is already used by the script, but could be used more aggressively to support less rigid coupling between the infrastructure and the systems. When discussing data, things are clearly defined (what is this value). Metadata can capture details not easily understood by having just the data (such as where did this value come from, or what error checks or value ranges were tested against this value, or where is this value present elsewhere in this data). This type of data, centrally managed, could provide a number of gains for generating documents and automating processes. Further, seeing a comprehensive view of the data and metadata could be valuable in making business decisions and clinical analysis. Metadata can also be used to drive jobs and processes, making system automation and configuration less painful.
To conclude
Data management is a task common to all clinical organizations. Supporting multiple vendors and simultaneously building scalable systems requires some attention to automation. The key benefits of understanding how to abstract your processes into definable tasks configurable via metadata is that the programming logic and business logic are separated. By compartmentalizing these two facets of the system, each can evolve without introducing undue strain on the other. The success of any new technology introduction depends heavily on understanding the needs of the user community and building a system to address the pain points of existing approaches. By automating data management through metadata, the laborious task of getting data to statistical programmers becomes greatly simplified and as such is exposed to a larger community than with any ad-hoc system. This creates a collaborative environment where data management, IT, applications development, and statistical programming could work together easily to get things done – and most importantly provide a common framework and therefore language for dealing with this task.
MaxisIT
At MaxisIT, we clearly understand strategic priorities within clinical R&D, and we can resonate that well with our similar experiences of implementing solutions for improving Clinical Development Portfolio via an integrated platform-based approach; which delivers timely access to study specific as well as standardized and aggregated clinical trial operations as well as patient data, allows efficient trial oversight via remote monitoring, statistically assessed controls, data quality management, clinical reviews, and statistical computing.
Moreover, it provides capabilities for planned vs. actual trending, optimization, as well as for fraud detection and risk-based monitoring. MaxisIT’s Integrated Technology Platform is a purpose-built solution, which helps Pharmaceutical & Life sciences industry by “Empowering Business Stakeholders with Integrated Computing, and Self-service Dashboards in the strategically externalized enterprise environment with major focus on the core clinical operations data as well as clinical information assets; which allows improved control over externalized, CROs and partners driven, clinical ecosystem; and enable in-time decision support, continuous monitoring over regulatory compliance, and greater operational efficiency at a measurable rate”.