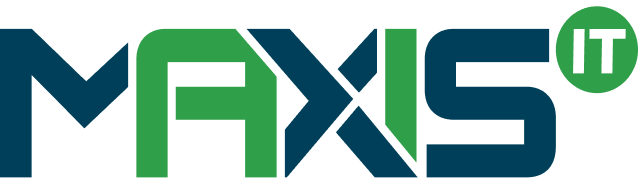
-
WHAT WE DO
- CLINICAL SOLUTIONS
- SERVICES
-
WHO WE ARE
-
RESOURCES
- CAREERS
- CONTACT US
This blog is the second in a 2-part series that discusses the increasing variety of data types and sources. The first installment described the increasing variability of sources, types, and formats. This blog focuses on the impact on the people, processes, and technology involved in clinical trials.
The variety, volume, and velocity of data coming form new sources in new formats and in new ways is changing the way the clinical research industry works. It is impacting the people, processes, and technologies that support clinical trials and motivating innovations across research organizations.
Technology
Digitization is working as a double-edged sword as it is helping to source more data than ever before, while wearable technology is creating more data than ever before. New data can provide unprecedented insights if clinical trial teams are using robust technologies. The variety of tech-enabled sources of clinical data include:
o IoT, wearables, and other patient generated data (e.g.: Home BP monitors),
o EHR, physiologic sensors, and apps,
o Lab tests, and Biomarkers,
o EDC systems, and mobile Healthtech,
o Real-World Evidence (RWE) Outcomes as data and images,
o Patient-reported outcomes, and patient diaries,
o Quality of life measures,
o Condition-specific or disease-specific registries
o Genomic data
Implementing technologies that quickly and accurately standardize and aggregate clinical trial data is a priority. Emerging technologies such as artificial intelligence (AI) and machine learning (ML) are revolutionizing the way teams approach the data lifecycle. Automation is essential to managing large quantities of variable data while also decreasing cycle times in an effort to shorten the time-to-market for new medicines.
People
Clinical data managers face an uphill task trying to fill gaps in data and ensuring its cleanliness to meet compliance requirements. When decentralized trials generate enormous amounts of data, the task of a data manager becomes significant, as they strive to ensure access to authoritative data. Data managers today need timely access to authoritative, standardized, and aggregated clinical trial operations data as well as patient data from site, study and portfolio level views. Today’s data managers are technically savy and use tools that support their innovative strategies for the collection, processing, and analysis of clinical data. They benefit from tools that flag any discrepancies as soon as they occur, in real-time. They manage their clinical trials using analytics and reporting for timely insights. They demand efficient trial oversight to address operational, quality, and compliance efforts.
With access to the right technology, Data Managers can reduce the time needed to aggregate all the clinical data across the data silos created by the disparate sources, minmizing manual reconciliations. In a process powered by artificial intelligence and machine learning, clinical data across an entire portfolio can be aggregated in real time, flagging risks and offering actionable insights. All clinical data can be integrated into a single data repository and act as a single-source of truth. Data can be sliced and diced to offer role-based dashboards, promoting proactive and corrective action and resolution, as required.
This combination of management skills and capabilities enables Data Managers to adhere to timelines and regulatory requirements. It also minimizes cost overruns in clinical trials by enabling in-time decision-making.
Process
To be reliable and accurate enough to drive decisions, data needs to be captured, controlled, verified, and reviewed appropriately. All these are highly complicated stages in the process of collecting the right data. This complexity is further exacerbated by the different data sources from which the data gets collected and the varying formats in which it gets reported. Technology today promotes interoperability, vetting the data from diverse sources and collating it to derive a cohesive, high-quality set of standardized data for analysis and reporting. The complexity resulting from new data sources and types is creating a greater need for more and deeper collaboration across traditional clinical research teams. This level of collaboration assumes fast access to accurate data. Collaborative processes built on a common, integrated data platform will ensure success.
Clinical research teams around the globe are adopting innovative new processes across their clinical trial portfolios. Modern clinical data platforms help to improve compliance and mitigate risks in clinical trials, using AI-powered analytics to provide actionable insights which accelerate the drug development process and shorten a drug’s time-to-market.
About MaxisIT
At MaxisIT, we clearly understand strategic priorities within clinical R&D, as we implement solutions for improving Clinical Development Portfolios via an integrated platform-based approach. For over 17 years, MaxisIT’s Clinical Trial Oversight System (CTOS) has been synonymous with timely access to study-specific, standardized, and aggregated operational, trial, and patient data, enabling efficient trial oversight. MaxisIT’s platform is a purpose-built solution, which helps the Life Sciences industry by empowering business stakeholders. Our solution optimizes the clinical ecosystem; and enables in-time decision support, continuous monitoring over regulatory compliance, and greater operational efficiency at a measurable rate.
This blog is the first in a 2-part series that discusses the increasing variety of data types and sources. The first installment describes the increasing variability of sources, types, and formats. The second installment discusses the impact on the people, processes, and technology involved in clinical trials.
Emerging technologies are enabling clinical research teams to address the speed at which high amounts of varied data are being evaluated in clinical trials, as myriad, decentralized sources bring in increasingly complex clinical trial data to them. The digital transformation brought by the COVID-19 pandemic emphasizes the need for effective strategies to access reliable, accurate data gleaned from decentralized trials. The clinical trials industry must leverage technology and implement automated solutions, which incorporate artificial intelligence and machine learning, to stay on course with trial timelines.
Considerations based on the Type of Data, and its Attributes:
Structured and Unstructured Data
Structured data is typically data that conforms to a pre-defined data model, like a spreadsheet with defined rows and columns. Unstructured data is variable and does not have a consistent underlying data model. Unstructured data includes data that is found within the text such as emails, documents, some survey results, presentations, and even social media posts. As we see more new sources of data coming directly from patients, we can assume that clinical research teams will need the capability to manage greater volumes of unstructured and structured data, as well as essential metadata that helps to understand and access the data.
Batch, Stream, Micro-batch Processing
Digitization enables new data to be operationalized quickly and prepares it for analysis. Data can be processed as it arrives, in real-time. It can also accumulate before being processed.
Batch processing runs on a set schedule, allowing data to accrue or reach a specific threshold before it is processed.
Stream processing is about processing data as soon as it arrives. This could be almost as soon as (or milliseconds after) it is generated if the aggregation happens in real-time. When we have data that is generated in a continuous stream, stream processing is the best option.
In micro-batch processing, processes are run on accumulations of data – typically a minute’s worth or less. This makes data available in near real-time.
Legacy Data
Drug sponsors have a growing need to convert legacy data into CDISC compliant formats, prior to submission to meet regulatory requirements if their submissions are to be accepted.
Data Quality & Cleansing
As noted previously, clinical data can come in from disparate sources in the form of a recordset, table, or database and numerous other unstructured formats. It’s important to have a processing and cleansing strategy for each data type to boost its consistency, reliability, and value. These processes vary for each type of data but must produce consistently accurate quality data. By detecting and correcting corrupt, incomplete, irrelevant, and inaccurate records early, clinical trial teams can get quicker access to higher quality data. This driving principle forms an essential and critical foundation for all data management strategies.
Results
Gone are the days when clinical research teams were using Excel sheets and SAS to collect and integrate clinical data. Clinical researchers are taking full advantage of the data available to them, using sophisticated, tech-enabled tools. As automated processes ingest data from disparate sources as soon as it gets generated, research teams get to access large volumes of high-quality data for analysis and to derive insights. This calls for technology with the capability to capture, organize, analyze, and report clinical trial data, or use new and improved visualizations to make better sense of the data and to enable research teams to derive greater insights than ever before.
About MaxisIT
At MaxisIT, we clearly understand strategic priorities within clinical R&D, as we implement solutions for improving Clinical Development Portfolios via an integrated platform-based approach. For over 17 years, MaxisIT’s Clinical Trial Oversight System (CTOS) has been synonymous with timely access to study-specific, standardized and aggregated operational, trial, and patient data, enabling efficient trial oversight. MaxisIT’s platform is a purpose-built solution, which helps the Life Sciences industry by empowering business stakeholders. Our solution optimizes the clinical ecosystem; and enables in-time decision support, continuous monitoring over regulatory compliance, and greater operational efficiency at a measurable rate.