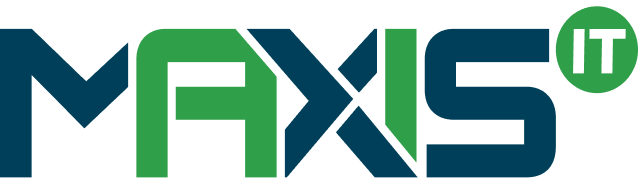
-
WHAT WE DO
- CLINICAL SOLUTIONS
- SERVICES
-
WHO WE ARE
-
RESOURCES
- CAREERS
- CONTACT US
What would you expect from a platform which offers to manage your clinical trials? Would you expect timely access to as authoritative, standardized and aggregated clinical trial operations data as well as patient data from site, study to portfolio level? Would you need efficient trial oversight via remote monitoring, statistically assessed controls, data quality management, clinical reviews, and statistical computing? Would you expect it to learn the patterns in the data and identify any discrepancies soon as they occur, in real-time?
If you don’t, it’s time you did. Yes, you heard that right. Demand more. Never settle.
Ensuring the Timely access to authoritative Data
After all, clinical trials are known to face issues with time and cost overruns. They could also fail to enroll on time, quality compliance, or low performance. Many even fail to clear the hurdles set by regulatory requirements. All this happens because the data was not accessible timely and required insight was not delivered to enable in-time decision making and issues were not identified or flagged in real-time and addressed appropriately.
Authoritative data is the sine-qua-non for any successful clinical trial. Only then would a trial stay within budget, meet its timelines and adhere to compliance requirements. There’s no disputing the fact that clinical data managers work very hard to ensure that their data is clean, but their task becomes superhuman as the data gets generated at tremendous rates and keeps on piling up. There’s always a risk of finding some gaps as some data usually goes missing. All these aspects make the task of a clinical data manager almost impossible to be done manually without the help of a tool.
How can technology help?
The market may be full of tools, but it’s important to ask if a tool has the required capabilities. It could help to improve the time taken to complete the task, beyond all recognition. It could aggregate all the clinical data in real time, across the data silos created by the disparate EDCs, CTMSs in use by the CROs and partners. It could offer a process powered by artificial intelligence and machine learning. It could go up the learning curve to quickly identify the trends in the data to flag any outliers and mitigate possible risks and manage the KPIs. It could offer actionable insights which support proactive action.
With the right technology, the data speaks to the mangers in various ways, like the following:
Who wouldn’t want to have a single tool which offers you all these capabilities, across your entire clinical trials portfolio?
Wake up call to Data Managers
Data managers need to refuse to be martyrs to their profession and be stuck with managing everything without the help of the right tool. Diversified host of eClinical systems only add to the issue, by creating siloed data and cannot offer an integrated view of all the trial data in real time. Technologically advanced tools like MaxisIT’s CTOS have been here for the last 17 years – with industry leading capabilities which make it possible for data managers to gain total oversight over their clinical trial portfolio data and enable them to take proactive action in real time. Data managers literally get into the driving seat when they adopt MaxisIT’s CTOS.
About MaxisIT
At MaxisIT, we clearly understand strategic priorities within clinical R&D, as they resonate well with our own experience of implementing solutions for improving Clinical Development Portfolio via an integrated platform. An ideal platform delivers timely access to study-specific as well as standardized and aggregated clinical trial operations as well as patient data, and allows efficient trial oversight via remote monitoring, statistically assessed controls, data quality management, clinical reviews, and statistical computing. Moreover, it provides capabilities for planned vs. actual trending, optimization, as well as for fraud detection and risk-based monitoring.
MaxisIT’s Clinical Trials Oversight System (CTOS) enables “data-driven digital transformation” by its complete AI enabled analytics platform. From data ingestion, processing, analysis to in-time clinical intelligence by establishing value of data. The CTOS empowers clinical stakeholders to mitigate risks and seize the opportunity in the most efficient manner at a reduced cost.
As discussed in Part 1 and Part 2 of AI in Clinical Trials, to process a large and continuously flowing stream of data, the pharma industry will need to employ an equally swift platform to ingest, standardize and manage the data, i.e. a holistic clinical data management platform. With the help of AI, MaxisIT’s Clinical Trial Oversight Platform ingests data from different sources, aggregates, and stores them into a repository. It also runs analytics without the need for coding and delivers actionable insights.
With improvements in electronic data capture, human errors in data capture will be eliminated or at the least be reduced drastically to enable instant integration with databases. Such seamless data management should reduce the amount of time and manual effort put into clinical data management processes.
AI will also help in reducing the overall burden of clinical data management by generating queries and reducing unnecessary and low-impact queries. This reduction in unnecessary queries will give clinical study stakeholders more time to concentrate on higher-value clinical tasks.
Modernize the clinical development process by integration with MaxisIT’s AI-based Clinical Trial Oversight platform
About MaxisIT
At MaxisIT, we clearly understand strategic priorities within clinical R&D, and we can resonate that well with our similar experiences of implementing solutions for improving Clinical Development Portfolio via an integrated platform-based approach; which delivers timely access to study specific as well as standardized and aggregated clinical trial operations as well as patient data, allows efficient trial oversight via remote monitoring, statistically assessed controls, data quality management, clinical reviews, and statistical computing. Moreover, it provides capabilities for planned vs. actual trending, optimization, as well as for fraud detection and risk-based monitoring.
MaxisIT’s Clinical Trials Oversight System (CTOS) enables “data-driven digital transformation” by its complete AI-enabled analytics platform from data ingestion, processing, analysis to in-time clinical intelligence by establishing the value of data; which empowers clinical stakeholders to mitigate risks or seize the opportunity in the most efficient manner at a reduced cost.
In Part-1 we discussed the problem statement and the focus areas for clinical development. We also concluded that quick access to relevant information decides the efficiency of a clinical trial. Let us now see how AI can help.
An end-to-end clinical data management platform powered by artificial intelligence is the right choice for streamlining, overseeing and managing trials in a coordinated way. With the help of a platform, study stakeholders can have defined role-based access and personalized views to data which makes it easy to monitor KPIs and stay on top of their tasks.
AI can predict risky sites
AI can predict risky sites by matching real-time data to historical benchmarks like drop-out rates and flag processes accordingly. Early red-flags makes it easy for Managers to keep the clinical trial on track and within budget. Such ability to find insights from big data would have been a tedious task for humans and would have consumed a lot of time but not for an AI platform. These insights would be on the dashboard by the time you make yourself a cup of coffee and this becomes instrumental in meeting the timelines and getting the drug faster to the market.
AI can tackle the need for Source Data Verification (SDV)
Source Data Verification (SDV) is one of the most important but time-consuming activities taken up during a trial. With all the constant manual checks to ensure compliance, it is also a major contributor to the cost of a trial. An AI-enabled platform can keep a tab on all types of inputs including data collected from patients, site performance data and completion metrics on Source Data Verification. The platform can run automated checks regularly to ensure that the processes comply with regulatory standards.
The platform makes it easy to manage data by standardizing the data from all sources into a singular regulatory format and storing them to be accessed as a singular source of truth. KPI’s can be predefined which will be tracked by the AI platform to identify potential protocol deviations and recommend course correction accordingly. It also records any deviations in the data and stores it to understand the pattern and detect much earlier in the future. After all, AI is a self-learner.
AI can collect and share information in real-time to improve the site and patient outcomes
AI provides users with access to data in real-time. This data is harmonized and is ready for analysis. The user can use a self-service visualization tool to keep a visual track on study milestones and checkpoints and immediately address any potential red flags. AI can also reduce patient dropouts by identifying the sites with access to the most relevant patient population.
According to visionary leader Steve Jobs, if one defines the problem correctly, that person almost has the solution. And here we are discussing AI as the bellwether solution to every business/operational challenge in this world. Throw in a few AI-related words and the conversation suddenly sounds futuristic and efficient. Sure, AI is already impacting us in various walks of life from online ads to streaming services to smart cars but what about business? In particular, drug development. Is AI ready to make its magic work for clinical trials? Let’s deep dive and try to find the answer.
How well have we defined the problem?
Challenges in patient recruitment, medication adherence, population health management, risk monitoring, data collection and aggregation for clinical trials are well documented. With the introduction of CDISC standards and ICH regulations, the process of recording and reporting data has undergone much-needed standardization. Yet as technology evolves, it adds new operational challenges. Here I am talking about new data sources, in particular, the rise in wearable technologies.
More and more patients are now using wearable devices to track health data thus creating a stream of continuous flowing data. This is a problem for an industry grappling with archaic systems and suboptimal processes for data management. The existing infrastructure is not capable of supporting big data and the processes require far too many human interventions to achieve the required level of efficiency. This is where cloud-based platforms and AI-driven workflow automation can make things better.
The Focus Areas of Clinical Trial Operations
To ensure efficient operations one must focus on the critical parameters of a clinical trial, which are
It all boils down to one crucial aspect, quick access to relevant information. Sounds easy but this is the deciding factor between the success and failure of a study. Currently, trial managers have to sift through tonnes of recorded information which requires a lot of time and patience. As a consequence, many of them miss crucial red flags leading to delays and in many cases, failure of the study itself.
In Part 1 and Part 2, we discussed the self-service analytics platform and its various components. In this part we will look into the important things to consider before implementing a self-service analytics platform. The historical way of representing clinical data includes spreadsheet driven models and custom SQL queries which not only increased development time and cost but also led to data quality issues. Self-service analytics has changed the way IT and business users collaborate to get insights from their information sources. Here are a few important considerations for implementing a self-service analytics platform.
Focusing on business impact
It is important for the leadership to identify how a self-service analytics platform can help them meet their business objectives. They should consider the impact on business functions and systems, involvement levels of various stakeholders, the capabilities required and the benefits that can be achieved. Self-service platforms represent the journey from retrospective reporting to the latest analytics capabilities (predictive analytics, AI). To be effective, a self-service analytics model should be flexible enough to accommodate and address the needs of various stakeholders and provide an easy path for them to achieve their business objectives.
Removing barriers to analytics adoption
Complex technology environment often limits the success of analytics efforts. In such environments, more than half of an analyst’s time is spent on overcoming analytics-related challenges such as collating scattered data across sources, improving data quality, and re-presenting insights to decision-makers. Moreover, dependency on IT staff for overcoming the above-mentioned challenges can delay the analytics process. A Self-service analytics platform can simplify the technology environment by automating data preparation tasks. This helps to shift the analysts’ focus on discovering and delivering valuable insights. Self-service analytics can also improve follow-through and responsiveness by automating and streamlining data provisioning and data distribution. Thus, it is important for Pharma companies to restructure processes and consider platforms that enable self-service analytics.
Implementing modern analytics architecture
Stakeholders of clinical trials need a flexible architecture to combine data from multiple sources and in multiple formats and then work with the combined dataset using their preferred analytic tools. New technology platforms can simplify tasks such as report generation and dashboard creation. Integrated platforms such as the MaxisIT’s Integrated Clinical Development Platform can streamline access to relevant data, automatically generate technical metadata, facilitate data preparation, and deliver consumable insights through front-end applications. The platform also offers advanced capabilities such as data discovery, data visualization, and artificial intelligence thus helping move analytics closer to end-users to impact business outcomes. Such an integrated platform positions the organization to take advantage of the convergence of other capabilities such as machine learning, digital assistants and the Internet of Medical Things.
Creating an Analytics governed and Data-driven strategy
Pharma companies can enhance their strategic decision making from the ground up by empowering stakeholders with on-demand insights about clinical trial operations. Doing so can help stakeholders make more timely decisions and course-corrections, often resulting in significant performance improvements. Self-service analytics users can benefit from capabilities such as automated data catalogs, common business rules, vetted algorithms, and business metadata that guide them to trusted analytics insights. When aligned with enterprise priorities and comprehensive data governance program, the organization can gain a repeatable, scalable framework that increases the agility and effectiveness of future analytics projects.
Keeping these considerations in mind can expedite the implementation of a self-service analytics program and help avoid many of the pitfalls that impede value realization.
About MaxisIT
At MaxisIT, we clearly understand strategic priorities within clinical R&D, and we can resonate that well with our similar experiences of implementing solutions for improving Clinical Development Portfolio via an integrated platform-based approach; which delivers timely access to study specific as well as standardized and aggregated clinical trial operations as well as patient data, allows efficient trial oversight via remote monitoring, statistically assessed controls, data quality management, clinical reviews, and statistical computing. Moreover, it provides capabilities for planned vs. actual trending, optimization, as well as for fraud detection and risk-based monitoring.
MaxisIT’s Integrated Technology Platform is a purpose-built solution, which helps Pharmaceutical & Life sciences industry by “Empowering Business Stakeholders with Integrated Computing, and Self-service Dashboards in the strategically externalized enterprise environment with major focus on the core clinical operations data as well as clinical information assets; which allows improved control over externalized, CROs and partners driven, clinical ecosystem; and enable in-time decision support, continuous monitoring over regulatory compliance, and greater operational efficiency at a measurable rate”.
In Part 1 we introduced self-service analytics and discussed what an ideal self-service analytics platform should accomplish. In this part, we will be discussing the various components of a modern technology platform that enables self-service analytics.
Data ingestion – In a clinical trial setting, both structured and unstructured data is available from an ever-expanding range of sources. These sources even include streaming data and data generated by connected devices. Such data has the potential to enhance insights. Self-service analytics is all about accessing, preparing, and analyzing disparate data from across the data sources. To succeed, pharma companies will require the technology that can ingest data from a variety of sources and be scalable enough to accommodate newer sources. The MaxisIT ecosystem has a platform designed to efficiently ingest and store large sets of structured and unstructured data from traditional sources of data as well as other applications. The platform makes this data available for real-time access.
Storage and preparation – This layer organizes and transforms data in a format suitable for further analysis. The rise of cloud-based technologies has made it possible to store large volumes of data in their native formats. Automated data preparation tools can be used to represent the native data in a format that allows scientists to derive analytical insights from them. These tools help analysts understand the data by exploring the range of values, the format of fields and the plausibility of the data captured. Data Visualization tools can further help in understanding the distribution of the data before deep diving into analysis. How does this help? The benefits are two-fold
Through automated data preparation, stakeholders can generate comprehensive metadata that supports and supports and confirms with the data governance standards of the regulatory authorities.
Data consumption – Users of clinical data have different data needs at different points in time. No one business intelligence tool can comprehensively address those needs. This is where the modern self-service analytics platform steps in to fill the void. Through a common semantic layer that represents data in a non-technical manner, stakeholders can bypass the underlying complex data environment and find what they need using common business parlance.
Data Governance – The data governance process is needed to manage the integrity, usability, and security of clinical data. The importance and relevance of data governance are growing with the expansion of the clinical data ecosystem which now includes data from sensors and connected devices. Governance processes should include details on how data is ingested, transformed, prepared, stored, presented, archived, shared, and protected. Standards and procedures should be developed to manage data access by authorized personnel and ensure ongoing compliance with regulations. The platform should support Master Data Management (MDM) by allowing IT and stakeholders to access a centrally managed business glossary, data dictionary, metadata, and reference data. Last but not least, data governance workflows with clear accountabilities should support how stakeholders exchange information and manage data assets.
Once an organization deploys a self-service analytics program, it should have an integrated platform in place with underlying capabilities and infrastructure components to promote adoption and end-user satisfaction. The platform should
The pharmaceuticals and lifesciences industry is undergoing transformation at an unprecedented scale mainly due to the regulatory, diminishing margins, growing amount of data and push for AI. One way to keep up with this pace of change is to create a robust analytics infrastructure that will help sponsors organizations to share information more efficiently and engage everyone involved to maximize value.
Most players in our industry are still laggards when it comes to leveraging the potential benefits of analytics due to lack of appropriate technology, processes, and required expertise. To make timely strategic decisions, decision-makers need easy access to actionable information. Success lies in overcoming the challenge of legacy systems and archaic processes.
A novel approach, which will enable users to access data faster without compromising on the security, is very much needed. To that end, modern self-service analytics can help users derive actionable insights by giving them an easier and timely access to data. Read on to know-how.
What is self-service analytics?
Self Service analytics (SSA) is vastly different from traditional business intelligence (BI). While tradition BI tools require background and expertise in statistical analysis and data mining, SSA helps clinical and business professionals to access data independently. It does so by automating data access, preparation, consumption, and analysis. In a self-service analytics environment, users can create and access specific datasets and reports on demand without the help of an IT resource.
An ideal self-service analytics platform should be able to
Gartner predicted that by 2020 self-service analytics will make up 80% of all enterprise reporting. While the prediction is accurate, it is disheartening to see that our pharmaceutical industry contributes to a large chunk of the 20% population who are still to adopt. Pharmaceutical companies need to restructure their analytics models to become more agile and successful. The ones who make the transition early are sure to reap the benefits.
By leveraging a modern analytics architecture and a platform for self-service analytics that can be scaled across the enterprise, these organizations would transcend from traditional reporting and business intelligence tools to automate data preparation and advanced analytics capabilities.
As clinical studies increase in complexity in a myriad of ways, a key question often asked is, “Is our ability to create complexity increasing faster than our ability to understand complexity?” This is an exciting time to be involved in the reporting of data and metrics on the performance of a clinical study. However, it is important to understand the principles of visual presentation of data to ensure that the information is accessible, actionable, not misleading and ultimately valuable for the end consumer of information
A multitude of roles are involved in a clinical trial such as Clinical Study Leader, Clinical Supply Managers, Statisticians, Country Managers, CRAs/Monitors, and Site Personnel. In product management terms, these roles are considered User Personas. A clear understanding of these roles and the activities they are required to perform by asking the question ‘what problems are you trying to manage?’ provides the framework for identifying the information they need to perform their jobs.
Also important is understanding the lifecycle of a clinical study which, at its broadest, can be considered inception, design, start-up, subject recruitment, study conduct, close out, and submission. These lifecycle steps should be used to influence when information is presented and how its prominence may change over time, depending on the study status. Delivering information that is relevant to the user in alignment with business processes will help ensure that metrics are actionable and used.
There are generally 4 types of metrics that one could measure in the clinical trial industry:
Anecdotally, it would appear that while there is much focus on metrics addressing both cycle time and timeliness, there are fewer that monitor quality and cost within the clinical trial industry. These four elements need to be in balance to ensure that doing something on time does not result in a lot of rework along the way. One of the metrics that is often discussed in Electronic Data Capture (EDC) solutions is the close out rate of queries (i.e., the time taken from when a query is raised until it is resolved). This is a cycle time metric. Although important in a trial, this is measuring rework (i.e., a lack of quality). This is an example of an ineffective resource investment as it does not materially contribute to the outcome of the trial. Conversely, focusing effort on reducing the number of queries raised has twice the impact as it improves quality AND reduces the amount of resources required to do the rework. Focusing in this area is an effective use of resource as it materially contributes to the outcome of the study.
It is essential that these key points remain at the forefront:
Having the right information readily accessible especially for data-intensive clinical trials at the right time and in a format that is easy to understand helps focus on what is important. Without focus it is easy to become distracted and dwell on issues that do not have a positive impact on objectives. Keeping these basic principles in mind when defining metrics and designing how to display them is essential in delivering metrics that are valued by the user.
MaxisIT with its cloud based integrated solution brings an opportunity to sponsor to improve oversight of clinical investigations by enabling standardization and storage of data, allowing integration with different EDC,CTMS, Safety, PV, Health Care, and document management systems.
Predictive analytics with dashboard for metrics, key performance indicators, key risk indicators, configurable thresholds, triggers, alerts, escalations and workflows to drive proactive risk mitigation and actionable outcomes are the major features associated with our solution, enabling sponsors to take timely decisions and reassess the monitoring strategy throughout the monitoring cycle.
Increasing clinical development costs for drugs has been a concern for industry over the years and multidirectional efforts have been made to lower these costs through more efficient study management. Since monitoring accounts for a substantial proportion of the total study costs, major focus is towards lowering the monitoring costs through the analysis of risks involved during a clinical drug development lifecycle.
Significant savings have been claimed through the use of modified site management; centralized and planned source document verification with only essential onsite source data verification emerging out of inconsistencies assessed through centralized risk based monitoring.
Monitoring is an essential element of clinical trials, ensuring quality and integrity of a clinical investigation. Monitoring uncovers potential problems such as data entry errors or missing data, assures that study documentation exists, assesses the familiarity of the site’s staff with the protocol and required procedures, and provides a sense of the overall quality of a site.
Post FDA’s final guidance and EMA reflections on Risk Based Monitoring, Industry is transitioning from routine visits to clinical sites and 100% Source Data Verification to risk-based approaches to monitoring. This helps sponsors to focus on critical data elements by practicing Centralized Monitoring and relying more on technological advancements thus reducing trial cost and time significantly.
Factors like central data collection systems, and real time data standardization and analytics are important to get a sense of the big picture in order to effectively perform risk-based monitoring. EDC and CTMS have made central data collection possible with higher level of data accuracy than that with traditional data collection methods.
Modern analytics tools and technologies are driving the emergence of centralized monitoring because they provide powerful insights into data. Considering the large scales of current clinical trials, accuracy and effectiveness is problematic with on-site monitoring. Large problems go unnoticed when data results are only skimmed through on-site monitoring practices
What do the authorities have to say?
The EMA emphasizes on the identification of potential risks and prioritization should commence at a very early stage in the preparation of a trial, as part of the basic design process. The concerns with trial and protocol design, design of data collection tools/instruments, the design of the monitoring and data management strategies and plans, including the relative role of centralized versus on-site activities and the data quality tolerances, and the design of record keeping for the study should be addressed within the framework of these dimensions, implementing a quality by design approach. Risk assessment and mitigation plans should be appropriately disseminated within the organization, regularly reviewed and updated when new information becomes available.
FDA recommends that each sponsor design a monitoring plan that is tailored to the specific human subject protection and data integrity risks of the trial. The monitoring plan should identify the various methods intended to be used and the rationale for their use. Monitoring activities should focus on preventing or mitigating important and likely sources of error in the conduct, collection, and reporting of critical data and processes necessary for human subject protection and trial integrity.
Sponsors should prospectively identify critical data and processes, perform a risk assessment to identify and understand the risks that could affect the collection of critical data or the performance of critical processes, and then develop a monitoring plan that focuses on the important and likely risks to critical data and processes. The guidance highlights the importance of documenting the monitoring plan after assessing the project risks and needs. It also recommends that sponsors analyze ongoing data to continuously assess and adjust the monitoring strategy.
This encourage sponsors to adopt strategies that reflect a risk-based monitoring approach using a combination of monitoring strategies and activities. The approach should emphasize focus on centralized monitoring by identifying critical elements and a plan to address data integrity risks. Several initiatives are underway to promote RBM paradigms and different methodologies are being suggested to achieve maximum out of the RBM approach.
MaxisIT has been constantly in pursuit of providing best innovative solutions to divergent requirements of pharmaceutical industry. With unique integrated clinical development platform and analytical capabilities, our solutions have provided sponsors great ease of work enabling them analyze disparate data sources and derive critical decision scenario on the fly. MaxisIT ’s Holistic and Flexible solution architecture offers complete solution for RBM approach in a real sense.
MaxisIT
At MaxisIT, we clearly understand strategic priorities within clinical R&D, and we can resonate that well with our similar experiences of implementing solutions for improving Clinical Development Portfolio via an integrated platform-based approach; which delivers timely access to study specific as well as standardized and aggregated clinical trial operations as well as patient data, allows efficient trial oversight via remote monitoring, statistically assessed controls, data quality management, clinical reviews, and statistical computing. Moreover, it provides capabilities for planned vs. actual trending, optimization, as well as for fraud detection and risk-based monitoring. MaxisIT’s Integrated Technology Platform is a purpose-built solution, which helps Pharmaceutical & Life sciences industry by “Empowering Business Stakeholders with Integrated Computing, and Self-service Dashboards in the strategically externalized enterprise environment with major focus on the core clinical operations data as well as clinical information assets; which allows improved control over externalized, CROs and partners driven, clinical ecosystem; and enable in-time decision support, continuous monitoring over regulatory compliance, and greater operational efficiency at a measurable rate”.
Post FDA’s final guidance on Risk Based Monitoring, Industry is transitioning from routine visits to clinical sites and 100% Source Data Verification to risk-based approaches to monitoring, focusing more on critical data elements by practicing Centralized Monitoring; relying more on technological advancements thus reducing trial cost and time significantly. The industry needs an out-of-the-box end to end solution that will cover all the bases for efficient risk based monitoring allowing business to stay agile and lean while making better and informed business decisions that will allow them to achieve faster and quality clinical drug development compliant to regulatory and assured cost savings
Data Integration
As technology is playing vital role in data collection during clinical trial conduction, multiple data sources like EDC, CTMS, PV, IVRS needs to be handled by sponsor. Before applying analytics on the data it is of utmost importance to place all data at single place. MaxisIT ’s unique data integration capability enables tool to communicate with disparate data sources. As integration is metadata driven it is highly configurable to sponsor specific metadata as well as standard metadata.
Source Data Validation (SDV)
Risk based monitoring emphasizes on selective source data validation in place of onsite 100% source data validation. Our solution offers seamless, real time data validation to extract discrepancies like missing data, duplicate records, data outliers, and inconsistent data. SDV engine also enables users to identify data fraudulence. This level of data validation increases data integrity and quality.
Data Standardization
Data standardization is the first step to ensure that your data is able to be used for analysis and shared across the regulatory. This establishes trustworthy data for use by other applications. Ideally, such standardization should be performed during data entry. If it is not done a comprehensive back end process is necessary to eliminate any inconsistencies in the data. Our standardization capability provides most comprehensive data standardization and transformations across the standards. Being a Metadata driven process it is flexible and configurable across different data standards. Its drag and drop utility makes data standardization easiest ever.
Analytics and Reporting
Effective risk based monitoring can be achieved using different statistics on disparate data related to ongoing trials. Many analytical tools are focusing their efforts on developing RBM oriented analytical engines with user friendly dashboards. Statistics being a functional entity limited to specific user group, configuration of complex statistical reports is never been a welcome step for end user. Data aggregation is imperative while handling disparate data for analytics. Our solutions provide metadata driven data aggregation which enables complex data analytics easier for user. It enables user to have cross functional report generation and better visibility through different data having linkage or dependencies.
MaxisIT ’s innovative analytics tool offers most user friendly operability and role based dashboard allowing users to have multiple reports like data driven visualizations, statistical reports and scenario modeling. Our analytics dashboard provides unique functionality of cross-functional drilldowns. Drilldown functionality allows user to navigate from one report to multiple another reports to understand depth of data and analyze root cause of issue. Similarly our unique entity of scenario modeling leverages understanding of complex cross-functional correlations and enables user to understand complex issue origins.
Monitoring Issue and Risk Management
Management of risks and issues emerged during central and onsite monitoring needs to be handled efficiently for faster resolution. Knowing the risks during monitoring execution and assessing the issues derived from central and onsite monitoring is centralized in our solution to allow the user to have easier navigation through monitoring interfaces and better visibility of all issues, action, and status with multiple levels of filters. User is able to generate monitoring reports based on the study, site, or other attributes by applying filters in our monitoring report generation interfaces. It also allows input of monitoring activities for onsite monitoring which updates central issue log. Our risk and issue log allows users to have an overview of complete status report and audit trails for all issues occurred during the study. All the reports generated are exportable in different formats like xls, pdf, png, jpg, html depending upon the report types. Trial conduction also contains a large amount of content management in form of multiple documents and forms. Our content management platform gives users a simplified content development solution where content development is highly organized with author reviewer workflow and features like reusability, resulting in cost-effective and quality content development.
Regulatory Compliance
MaxisIT ’s solution is highly compliant to different global regulatory standards like 21 CFR part 11 or CSV guidelines by EMA etc. Central and highly secured data storage adds to the completeness of the solution where complete organizational usability of solution is through its single sign-on, role-based user access facilities making it a highly reliable, scalable and complete solution for RBM.
MaxisIT
At MaxisIT, we clearly understand strategic priorities within clinical R&D, and we can resonate that well with our similar experiences of implementing solutions for improving Clinical Development Portfolio via an integrated platform-based approach; which delivers timely access to study specific as well as standardized and aggregated clinical trial operations as well as patient data, allows efficient trial oversight via remote monitoring, statistically assessed controls, data quality management, clinical reviews, and statistical computing. Moreover, it provides capabilities for planned vs. actual trending, optimization, as well as for fraud detection and risk-based monitoring.
MaxisIT’s Integrated Technology Platform is a purpose-built solution, which helps Pharmaceutical & Life sciences industry by “Empowering Business Stakeholders with Integrated Computing, and Self-service Dashboards in the strategically externalized enterprise environment with major focus on the core clinical operations data as well as clinical information assets; which allows improved control over externalized, CROs and partners driven, clinical ecosystem; and enable in-time decision support, continuous monitoring over regulatory compliance, and greater operational efficiency at a measurable rate”.