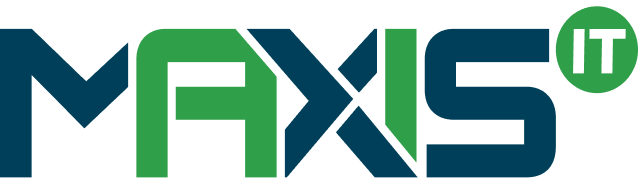
-
WHAT WE DO
- CLINICAL SOLUTIONS
- SERVICES
-
WHO WE ARE
-
RESOURCES
- CAREERS
- CONTACT US
In Part 1 we introduced self-service analytics and discussed what an ideal self-service analytics platform should accomplish. In this part, we will be discussing the various components of a modern technology platform that enables self-service analytics.
Data ingestion – In a clinical trial setting, both structured and unstructured data is available from an ever-expanding range of sources. These sources even include streaming data and data generated by connected devices. Such data has the potential to enhance insights. Self-service analytics is all about accessing, preparing, and analyzing disparate data from across the data sources. To succeed, pharma companies will require the technology that can ingest data from a variety of sources and be scalable enough to accommodate newer sources. The MaxisIT ecosystem has a platform designed to efficiently ingest and store large sets of structured and unstructured data from traditional sources of data as well as other applications. The platform makes this data available for real-time access.
Storage and preparation – This layer organizes and transforms data in a format suitable for further analysis. The rise of cloud-based technologies has made it possible to store large volumes of data in their native formats. Automated data preparation tools can be used to represent the native data in a format that allows scientists to derive analytical insights from them. These tools help analysts understand the data by exploring the range of values, the format of fields and the plausibility of the data captured. Data Visualization tools can further help in understanding the distribution of the data before deep diving into analysis. How does this help? The benefits are two-fold
Through automated data preparation, stakeholders can generate comprehensive metadata that supports and supports and confirms with the data governance standards of the regulatory authorities.
Data consumption – Users of clinical data have different data needs at different points in time. No one business intelligence tool can comprehensively address those needs. This is where the modern self-service analytics platform steps in to fill the void. Through a common semantic layer that represents data in a non-technical manner, stakeholders can bypass the underlying complex data environment and find what they need using common business parlance.
Data Governance – The data governance process is needed to manage the integrity, usability, and security of clinical data. The importance and relevance of data governance are growing with the expansion of the clinical data ecosystem which now includes data from sensors and connected devices. Governance processes should include details on how data is ingested, transformed, prepared, stored, presented, archived, shared, and protected. Standards and procedures should be developed to manage data access by authorized personnel and ensure ongoing compliance with regulations. The platform should support Master Data Management (MDM) by allowing IT and stakeholders to access a centrally managed business glossary, data dictionary, metadata, and reference data. Last but not least, data governance workflows with clear accountabilities should support how stakeholders exchange information and manage data assets.
Once an organization deploys a self-service analytics program, it should have an integrated platform in place with underlying capabilities and infrastructure components to promote adoption and end-user satisfaction. The platform should
7 Feb 2018
10 Jun 2019